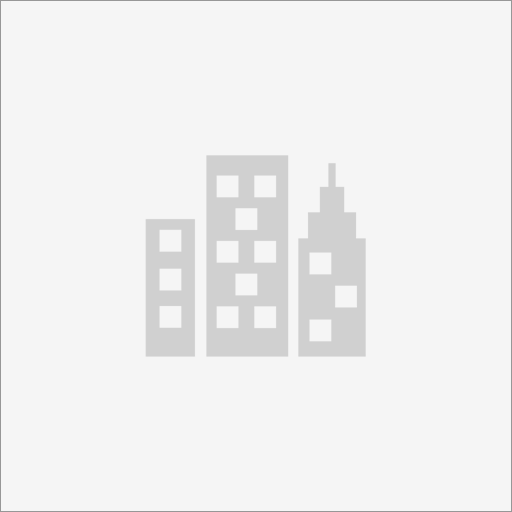
University of Sheffield
nearmejobs.eu
Health monitoring and predictive maintenance (HMPM) are crucial for preventing unexpected equipment failures and reducing maintenance costs. The main goal of the project is to explore data-driven methods including machine learning (ML) and artificial intelligence (AI) techniques, to develop predictive HMPM tools that can diagnose, detect, and predict faults in machinery components and subsystems. This helps in avoiding machinery shutdowns and minimising maintenance costs. One of the interests is to develop interpretable fault diagnosis and prognosis (iFDP) models, which can not only provide accurate detection and diagnosis but also provide insights into why and how a particular fault is predicted, so as to enhance the robustness, reliability and trustworthiness of the relevant decision-making. Experimental datasets, recorded on numerous industrial machines and processes (e.g., wind farms, wind turbines, rolling bearing rigs, planetary gearboxes, aircraft engines, drive and bearing, and chemical processes), are available for use in testing the performances of the developed models.
To help us track our recruitment effort, please indicate in your email – cover/motivation letter where (nearmejobs.eu) you saw this posting.