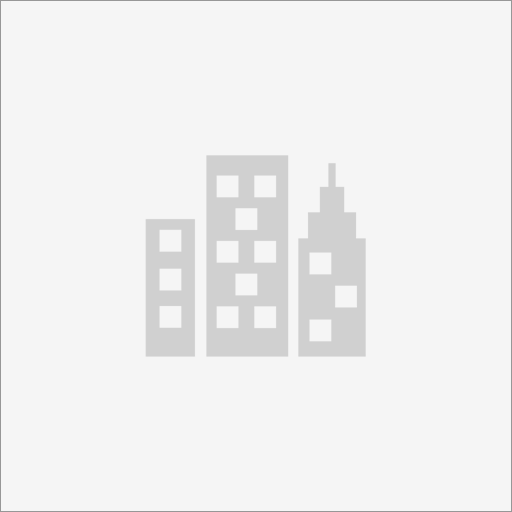
University of Birmingham
nearmejobs.eu
Oral cancer is a global health problem with little improvement in survival rates, often attributed to late diagnosis. One of the obstacles is the inability to reliably predict malignant transformation in a range of disorders with an increased risk of developing malignancy termed oral potentially malignant disorders (OPMDs).
The histological changes associated with OPMDs are described as ‘oral epithelial dysplasia’ (OED), which are subjectively graded, resulting in low predictive value. Histopathological findings of OED indicate that the lesion has, statistically, a risk of malignant transformation, but it cannot be used for a reliable prediction. The problems with OED grading arise from the subjective evaluation of the morphological parameters used to define OED in addition to the lack of calibration and the absence of validated criteria that are important for predicting malignant transformation.
This highlights an urgent need for objective, reproducible grading systems based on morphological and biological criteria predictive of malignant transformation.
Aim:
This multidisciplinary project aims at addressing diagnostic difficulties of grading OED through combining digital pathology with machine learning to objectively detect changes associated with disease progression, thus identifying features that reliably predict malignant change.
Objectives:
- Evaluate histo-morphological features diagnostic of OED using digital pathology to automate their assessment and use machine learning to identify the parameters capable of distinguishing different diagnostic entities.
- Apply deep learning models to histopathological images to identify their ability to classify them and identify parameters capable of differentiating diagnostic groups.
- Incorporate the identified parameters in a modified OED grading system.
- Validate the modified OED grading system and test its predictive ability by evaluating retrospectively transformed and non-transformed OED cases.
Methods:
Whole slide scans of histopathological samples from normal, OED and squamous cell carcinoma will be analysed using open source imaging packages QuPath and ImageJ. Deep learning models based on Convolutional Neural Networks (CNN) or Transformer architecture will be applied to the images to test their ability to classify and identify parameters that differentiate diagnostic groups.
A modified OED classification system will be developed based on the identified parameters and tested on an independent cohort stratified into progressive and non-progressive OED cases.
Significance:
This study will demonstrate the feasibility of incorporating digital pathology and machine learning into the existing pathology workflows using standard tissue sections. The findings will inform the development of robust OED grading systems, capable of predicting malignant transformation allowing early detection of malignancy and ultimately improving patient outcomes.
You can find more information here: 125th Anniversary Scholarships for Black British Researchers – University of Birmingham
To help us track our recruitment effort, please indicate in your email – cover/motivation letter where (nearmejobs.eu) you saw this posting.