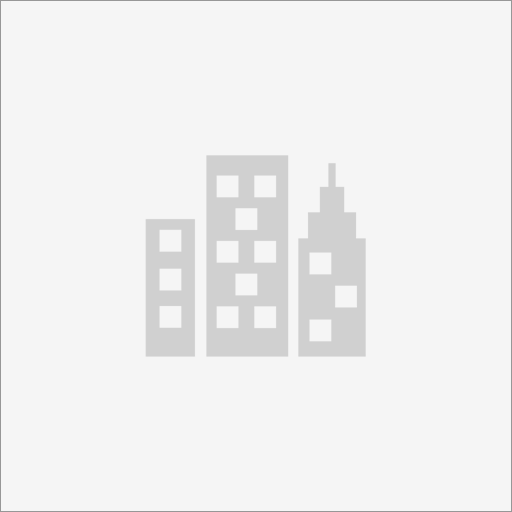
University of Leicester
nearmejobs.eu
GTA funded PhD studentship in Computing
Highlights
- To investigate novel AI models for producing automated digital twins with embedded physics in the learning process
- To investigate digital twins that learn physics from the data
- To investigate self-learning digital twins that can learn from the operational environment and can evolve as a result of new observations
Project
This is a collaborative research project between the University of Leicester, BT and Bloc Digital. BT will offer the expertise and data in monitoring large scale infrastructure projects such as 5G deployment and network planning. Bloc Digital will offer expertise in virtual and augmented reality and its use in producing digital twins. University of Leicester will research and develop next generation AI algorithms that can produce Physics informed digital twins in real time from vast amounts of data using HPC and in-memory Clouds.
The ability to visualize a system outcome before it happens is extremely valuable and digital twins enable this to happen using virtual reality models. Using digital twins, engineers can design better products while users can see final products pre-production, ultimately saving everyone time and resources.
Currently it takes weeks and months to produce digital twins because the process is manual and time consuming. This project aims to investigate an automated digital twin model that can learn physics from medical, engineering as well as infrastructure data and iteratively evolve as new data and insight become available over time. This will necessitate creation of new physics informed AI models and algorithms that can learn physics from engineering models with minimum computational cost. This will require embedding mathematical and physics models into the neural networks to govern the learning process or in other cases will require learning physics laws from the observation data. In both cases, this will require to build a framework for embedding physics
models into neural networks for end-to-end training on observation data. The resulting digital models will be put into practice to generate data that will be realistic emulation of the experimental environment and will be used to self-learn and optimize the digital twins to represent evolving functionality in the physical world.
These models and algorithms should have the capability to exploit high performance computing for real time model execution for large scale immersive design and analytics. Dynamically integrating data, algorithms & models will need careful understanding of geometrical and system models to minimise the computational burden. To overcome this problem, we will use high performance in-memory systems where we can store the components of the models in a distributed shared memory while the algorithms are processing the data for their models.
Enquiries to project supervisor Professor Ashiq Anjum [email protected] or [email protected]
Further details and application advice at https://le.ac.uk/study/research-degrees/funded-opportunities/cms-gta
To help us track our recruitment effort, please indicate in your email – cover/motivation letter where (nearmejobs.eu) you saw this posting.