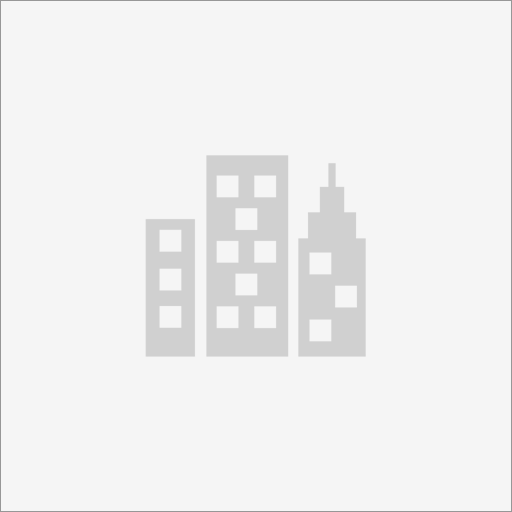
University of Sheffield
nearmejobs.eu
Digital twins are an emerging tool for the modelling and managing of structures. Research has focussed on developing models of whole structures, however, sufficient modelling accuracy and robustness is often achieved only for parts of structures or components. The complexity of such interactions may render traditional physical-modelling approaches insufficient. Similarly, the interaction between physical domains, such as fluid dynamics and structural dynamics, makes the modelling of the interface between the domains quite challenging. To tackle such problems, the current project will focus on developing data-driven methods to learn such interactions. Machine learning offers solutions to quite complex problems and learning the interactions between structural components and domains is one of them. Using data acquired from structures, machine-learning techniques will be integrated with existing knowledge regarding the individual components and domains and will offer a comprehensive modelling approach to structural dynamics. The developed machine-learning models will be integrated with existing validated models of components or domains and will be used to learn the interaction between them, aiming at increasing the accuracy and robustness of the whole-system model.
The project aims at evaluating the performance of different machine-learning methods for the learning the desired interactions and for defining digital twins of structures. The methods may span from simple techniques to more complicated deep-learning techniques and the main objective is to integrate different types of data and even various types of models in the inference procedure. The case studies of the project will be created using data from structures tested in the laboratory, e.g. available data from a Hawk aircraft, and from operational structures. The modelling technologies which will be developed will bridge the gap between modelling individual components and modelling whole structures, which is a significant barrier in developing large-scale digital twins.
During this project, you will gain significant experience in:
● Structural modelling
● Signal processing for structural modelling
● Analysing datasets from data acquired from structures
● Applying machine learning techniques for structural digital twins
● Presentation skills
● Working in a multidisciplinary/international environment
Requirements:
Education: A very good 4-year/master degree in Mechanical, Aeronautical, Marine, Civil, Chemical Engineering, Computer Science, Applied Mathematics or Physics (at least a UK 2:1 honours degree).
The successful candidate will work under the supervision of Dr George Tsialiamanis and will be part of a vibrant team of PhD students and RAs, the Dynamics Research Group (https://drg.ac.uk/, https://lvv.ac.uk/). The group includes people working in different areas of structural dynamics and machine learning and provides the opportunity to its members to define their own path in research and at the same time to collaborate with other members.
To help us track our recruitment effort, please indicate in your email – cover/motivation letter where (nearmejobs.eu) you saw this posting.