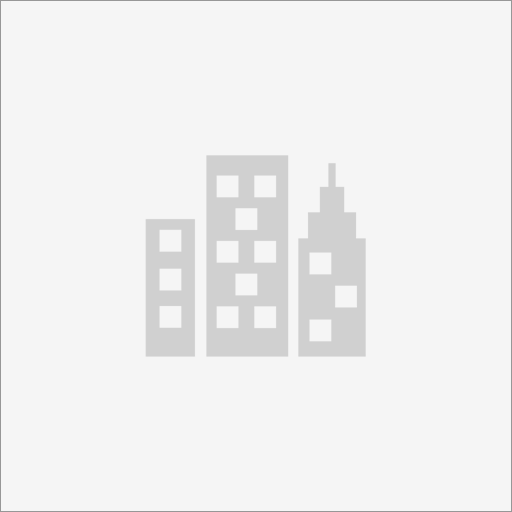
The University of Manchester
nearmejobs.eu
We are pleased to announce a highly competitive PhD opportunity in statistics with a focus on advanced machine learning techniques for Big Data analysis. This project is ideal for candidates eager to contribute to the theoretical development of statistical learning, with a particular emphasis on clustering methods, high-dimensional data reduction, and robust inference in healthcare settings. A key component of the research will involve integrating machine learning with traditional statistical paradigms, such as longitudinal modeling and survival analysis, to enhance predictive accuracy and uncover hidden patterns within large-scale datasets.
Objectives
(I) Develop advanced machine learning methodologies tailored for Big Data analysis, with a focus on clustering techniques, high-dimensional data reduction, and robust inference.
(II) Enhance existing statistical learning frameworks by integrating machine learning approaches with traditional statistical methods, such as longitudinal modeling and survival analysis, to improve predictive accuracy.
(III) Identify latent structures in large-scale datasets by leveraging scalable clustering algorithms.
(IV) Design computationally efficient algorithms capable of addressing challenges such as noise, missing data, and heterogeneity in Big Data.
(V) Validate the proposed methodologies to ensure their practical utility and generalizability, with a particular emphasis on applications in Electronic Health Records (EHRs) and other high-dimensional datasets.
Key Research Areas
The successful candidate will develop advanced machine learning techniques for healthcare Big Data, with a focus on clustering methods, high-dimensional data reduction, and robust statistical inference. The research will focus on creating scalable clustering algorithms to identify hidden structures in Electronic Health Records (EHRs) and other large datasets, as well as dimensionality reduction techniques to enhance model interpretability and performance.
A key aspect of the project will involve integrating machine learning techniques with traditional statistical approaches, such as longitudinal modeling and survival analysis, to improve predictive accuracy. The work will also focus on creating computationally efficient algorithms that can manage noise, missing data, and heterogeneity commonly found in healthcare datasets. Throughout the project, the candidate will validate these methodologies to demonstrate their practical utility and generalizability in Big Data analytics.
What We Offer
1. An opportunity to conduct pioneering research with real-world industrial applications.
2. Access to state-of-the-art facilities and resources.
3. A supportive and collaborative research environment.
4. Expert guidance and mentorship from leading professionals in the field.
5. Join us on a transformative journey where your passion for statistics, machine learning, and Big Data analysis will shape the future of data-driven research.
The project is a part of ongoing collaboration between researchers from University of Manchester and University of Dundee.
Eligibility
Applicants should have, or expect to achieve, at least a 2.1 honours degree or a master’s (or international equivalent) in a relevant science or engineering related discipline.
Essential attributes:
- MSc in Statistics, Mathematics, or allied areas (with a substantial background in statistics).
- Skill in R programming (substantiated by curriculum taken and/or projects).
- Proficiency in oral & written communication in English.
Desired:
- Evidence of interest in research
- Experienced or keen to learn advanced machine learning techniques.
The proposed start date for this project is January 2025.
Funding
At Manchester we offer a range of scholarships, studentships and awards at university, faculty and department level, to support both UK and overseas postgraduate researchers applying for competition and self-funded projects.
For more information, visit our funding page or search our funding database for specific scholarships, studentships and awards you may be eligible for.
Before you apply
We strongly recommend that you contact the supervisor, Dr Madhu Bhattacharjee, for this project before you apply.
Email at [email protected] [email protected]
How to apply
Apply online through our website: https://uom.link/pgr-apply-fap
When applying, you’ll need to specify the full name of this project, the name of your supervisor, if you already having funding or if you wish to be considered for available funding through the university, details of your previous study, and names and contact details of two referees.
Your application will not be processed without all of the required documents submitted at the time of application, and we cannot accept responsibility for late or missed deadlines. Incomplete applications will not be considered.
After you have applied you will be asked to upload the following supporting documents:
- Final Transcript and certificates of all awarded university level qualifications
- Interim Transcript of any university level qualifications in progress
- CV
- Supporting statement: A one or two page statement outlining your motivation to pursue postgraduate research and why you want to undertake postgraduate research at Manchester, any relevant research or work experience, the key findings of your previous research experience, and techniques and skills you’ve developed. (This is mandatory for all applicants and the application will be put on hold without it).
- Contact details for two referees (please make sure that the contact email you provide is an official university/work email address as we may need to verify the reference)
- English Language certificate (if applicable)
If you have any questions about making an application, please contact our admissions team by emailing [email protected].
Equality, diversity and inclusion is fundamental to the success of The University of Manchester, and is at the heart of all of our activities. We know that diversity strengthens our research community, leading to enhanced research creativity, productivity and quality, and societal and economic impact.
We actively encourage applicants from diverse career paths and backgrounds and from all sections of the community, regardless of age, disability, ethnicity, gender, gender expression, sexual orientation and transgender status.
We also support applications from those returning from a career break or other roles. We consider offering flexible study arrangements (including part-time: 50%, 60% or 80%, depending on the project/funder).
To help us track our recruitment effort, please indicate in your email – cover/motivation letter where (nearmejobs.eu) you saw this posting.