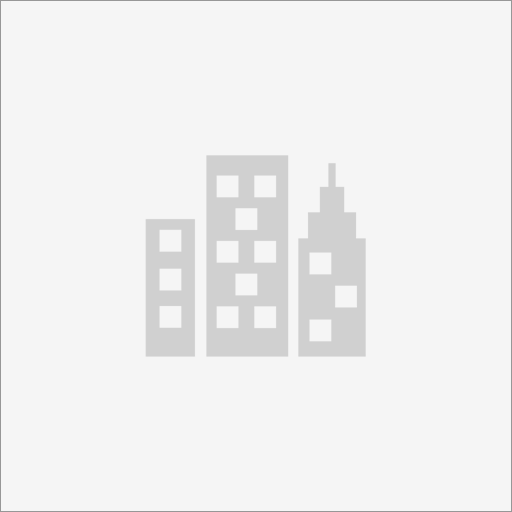
University of Reading
nearmejobs.eu
Background: Controlled environment (CE) facilities, such as glasshouses, play a vital role in UK agriculture, producing crops year-round and supporting research in plant sciences. However, seasonal variations, such as changes in light and temperature, still affect productivity and research outcomes. Current methods to mitigate these effects are often expensive, relying on advanced infrastructure or costly energy inputs. This project explores an innovative alternative: using artificial intelligence (AI) to analyse and adjust for seasonal impacts on plant growth, making controlled environments more efficient and cost-effective.
Research Aims: The project aims to understand how seasonal changes affect plant growth and to develop AI-driven strategies to predict and mitigate these effects. By combining advanced imaging, environmental data, and machine learning, the research seeks to create models that help plants thrive regardless of the season.
What the Student Will Do: As part of this cutting-edge research project, the student will gain hands-on experience at the forefront of plant science and artificial intelligence (AI). Working across world-class facilities, they will:
1. Master Advanced Imaging and Data Collection Techniques
They will use high-tech tools, including RGB cameras and other remote sesning/imaging systems, to capture detailed plant traits such as size, colour, and health. They will also gather environmental data (e.g., temperature, relative humidity, light) to build comprehensive datasets. This will provide valuable skills in experimental design, imaging technologies, and standardized data collection protocols.
2. Learn AI and Machine Learning Applications
The student will dive into machine learning, training neural networks to identify patterns in plant growth. They will learn to develop AI models that can predict crop performance and adapt to seasonal challenges. This hands-on exposure to data science tools will open doors to diverse career paths in agri-tech, research, and beyond.
3. Integrate Multimodal Datasets
By combining imaging, environmental, and phenotypic data, the student will co-register diverse datasets, transforming them into actionable insights. This interdisciplinary skill is in high demand and applicable to both research and industry roles.
4. Test Real-World Applications
They will validate AI predictions with traditional methods, comparing results to assess reliability. They will also evaluate the cost-effectiveness of AI-driven strategies, learn how to conduct impactful cost-benefit analyses and present findings to stakeholders.Working at the intersection of biology and technology, the student will develop a deep understanding of plant-environment interactions, cutting-edge AI tools, and practical problem-solving in agriculture. Beyond technical expertise, they will build transferable skills in critical thinking, communication, and collaboration.
Training opportunities: The student will receive comprehensive training to equip them with thein technical, analytical, and professional skills required for this multidisciplinary project.
1. University-Based Training: The student y will undergo training in experimental design, plant phenotyping, and data analysis techniques, utilizing cutting-edge imaging systems and analytical tools. Core courses in artificial intelligenceAI and machine learning will provide foundational knowledge in deep learning and neural network development.
2. Industry Training: The student y will engage directly with cutting-edge industry practices during periods of trainingtheir placement at Syngenta’s Jealott’s Hill research campus, one of Europe’s premier agricultural R&D facilityties. Here, tThey will actively contribute to experiments using state-of-the-art controlled environment systems to assess plant responses to treatments like (e.g. herbicides and growth regulators) across seasonal cycles and
3. Interdisciplinary Collaboration: Through collaborations with experts at Aberystwyth, Cranfield, and Syngenta, the student will gain insights experience in participating and managing into multidisciplinary approaches projects. Training will be available to strengthen communication, project management and other transferable skills, as well as programming and statistical skills.to solving agricultural challenges, including environmental data integration and cost-benefit analyses.
4. Upskilling Opportunities: Workshops and online courses will be available to strengthen programming, statistical, and communication skills, ensuring the student is well-prepared for both academic and non-academic careers. This well-rounded training program prepares the student to thrive in the rapidly evolving field of agri-tech.
Project supervision style: John Doonan will act as the lead supervisor, with weekly 1:1 meetings to provide consistent guidance. The student will also participate in our weekly lab group meetings for broader discussions and lead these on a regular basis. The student will have a monthly on line meeting with the supervisory team from Cranfield and Syngenta. Feedback on drafts and reports will be provided in line with normal University practice (within a max of two weeks for written work; same day for presentations).
Student profile: Candidates with strong quantitative or computational backgrounds, an interest in solving real-world agricultural problems, and a willingness to learn plant science would be well-suited for this project. While specific prior expertise is not required, enthusiasm for learning interdisciplinary skills and a proactive approach to tackling complex data integration challenges are the key prerequisites.
Skills in data analysis and computational methods, particularly familiarity with programming languages such as Python or R for working with machine learning and statistical models would be advantageous.
A foundational understanding of artificial intelligence or machine learning concepts is desirable but not mandatory, as training will be provided. Basic knowledge of plant biology or controlled environment agriculture is advantageous, particularly an understanding of phenotyping and plant-environment interactions, and the role of sensors in monitoring plant responses.
Other information: Syngenta will provide additional support for accommodation costs for all work conducted at the Jealotts Hill Research Campus. I
How to Apply: Applications will be by an online application form only. Do not send CVs. Please go to the FoodBioSystems website to see guidance to applicants, information on academic and funding eligibility and language proficiency.
Equality Diversity and Inclusion: The FoodBioSystems DTP is committed to equality, diversity and inclusion. Our actions to promote diversity and inclusion are detailed on the FoodBioSystems DTP website and include: Offering reasonable adjustments at interview for shortlisted candidates who have disclosed a disability or specific learning difference; Guaranteed interview and applicant mentoring schemes for applicants, with UK home fees status, from eligible under-represented ethnic groups. These are opt-in processes.
To help us track our recruitment effort, please indicate in your email – cover/motivation letter where (nearmejobs.eu) you saw this posting.