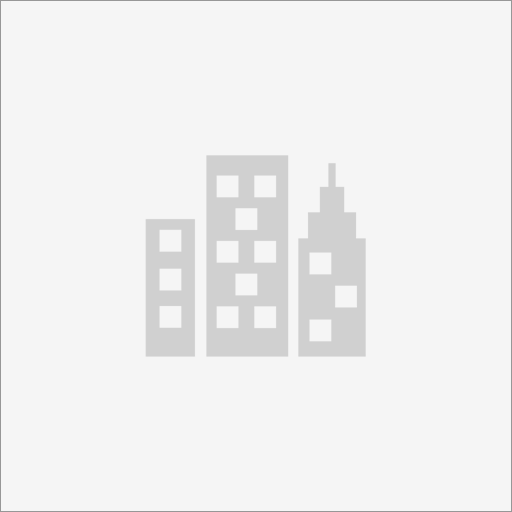
University of Leicester
nearmejobs.eu
GTA funded PhD studentship in Computing
Highlights
- Use smartphone and wearable technology for real-time monitoring and tailoring of physical activity rehabilitation programmes.
- Advancing disease management through predictive analytics based on physical activity data and machine learning.
- Spearheading interdisciplinary collaboration with the Biomedical Engineering Research Group, the Physical Activity and Rehabilitation Group and the Leicester Lifestyle & Health Research Group in order to merge state-of-the-art machine learning and software engineering with clinical physiotherapy insights.Proe
Project
In today’s fast-paced world, the journey from injury to recovery or managing multiple chronic conditions is unique for everyone, requiring more than a one-size-fits-all approach. Foreseeing a near-term future where your smartphone and wearable devices become your personal physiotherapists, providing real-time, custom-tailored guidance that adapts to your body’s needs and progress. With the rise of mobile health (mHealth) technologies, we have an unprecedented opportunity to transform rehabilitation practices. By combining the precision of biomechanical analysis with the accessibility of smartphones, this research aims to unlock new avenues for personalised healthcare. By doing so, we not only aim to significantly enhance the effectiveness of rehabilitation programmes but also empower individuals to take care of their recovery journey, making health management more intuitive, efficient, and aligned with the rhythm of their daily lives. This interdisciplinary collaboration seeks not only to refine rehabilitation practices but also to democratize access to personalised healthcare solutions, leveraging the ubiquity of mobile technology.
Research objectives:
Objective 1 involves the use of Theia 3D technology to identify key movement impairments in individuals with multiple long-term conditions (MLTCs) and functional limitations. This step will establish an effective understanding of the biomechanical challenges faced by patients, serving as a foundation for subsequent analysis.
Objective 2 focuses on determining whether these identified movement impairments can be accurately detected using accelerometer data, employing machine learning techniques. This innovative approach aims to bridge the gap between complex biomechanical data and accessible, real-time monitoring tools.
Objective 3 is dedicated to the development of a mobile application that uses accelerometer data to assess the degree of functional limitations. This app will provide tailored rehabilitation feedback, empowering patients to engage in personalised exercises that align with their specific needs and recovery progress.
Methodology:
This research will be inherently interdisciplinary, blending expertise in software engineering, specifically model-based software development and mHealth app design, machine learning with inertial measurement unit (IMU) sensor data, with clinical knowledge in physiotherapy. It will employ iterative development and validation of algorithms for both rehabilitation programme tailoring and disease flare-up prediction, with consistent input and validation from clinical collaborators to ensure applicability and effectiveness.
This project leverages the synergistic capabilities of two sophisticated systems: a traditional biomechanics motion tracking system (Biomedical Engineering Group) and Theia3D (Physical Activity Rehabilitation Group), a cutting-edge markerless motion capture technology. The integration of these systems provides a reliable framework for the analysis of high-fidelity, detailed human movement data. The traditional biomechanics motion tracking system, with its robust and precise capture of biomechanical movements, lays the groundwork for initial model development. It offers a reliable foundation for the creation and preliminary refinement of algorithms designed to interpret accelerometer data from wearable and smartphone devices.
Simultaneously, Theia3D enhances this process by serving as a benchmark for shaping, validating and further refining these algorithms. Its advanced markerless motion capture capabilities allow for the collection of movement data without the constraints of markers or specialized equipment, offering a more natural and comprehensive dataset. By recording movements concurrently with both Theia3D and the wearable devices, researchers can perform a nuanced comparison of the datasets. This comparison is crucial for assessing the precision and reliability of the accelerometer data against the high-resolution 3D movement data captured by Theia3D.
This dual-system approach ensures that the algorithms developed for interpreting accelerometer data are not only based on accurate and trustworthy information but are also refined to a degree of precision that reflects the complexities and subtleties of human movement. The integration of data from both the traditional biomechanics motion tracking system and Theia3D into the algorithmic refinement process is pivotal, as it guarantees that the rehabilitation programmes and interventions derived from this research are informed by a comprehensive understanding of movement, thereby enhancing the personalisation and effectiveness of patient care.
Impact for PhD Candidates: Engaging in this project allows PhD candidates to be at the forefront of melding cutting-edge technology with acute clinical needs. They will acquire a unique blend of skills in data science, software engineering, and clinical research application, positioning them as leaders in the emerging field of digital health. This project not only offers the opportunity to contribute significantly to improving patient care and disease management but also to blaze a trail in an interdisciplinary research area ripe with possibilities.
Enquiries to project supervisor Dr Artur Boronat [email protected] or [email protected]
Further details and application advice at https://le.ac.uk/study/research-degrees/funded-opportunities/cms-gta
To help us track our recruitment effort, please indicate in your email – cover/motivation letter where (nearmejobs.eu) you saw this posting.